The Power of Modeling
Perspectives | Aug 7, 2024
We use models as a powerful tool to help us understand and answer complex questions about the Gulf of Maine's ecosystems and climate. The information we learn using models is crucial for decision-makers. Read on to learn more.
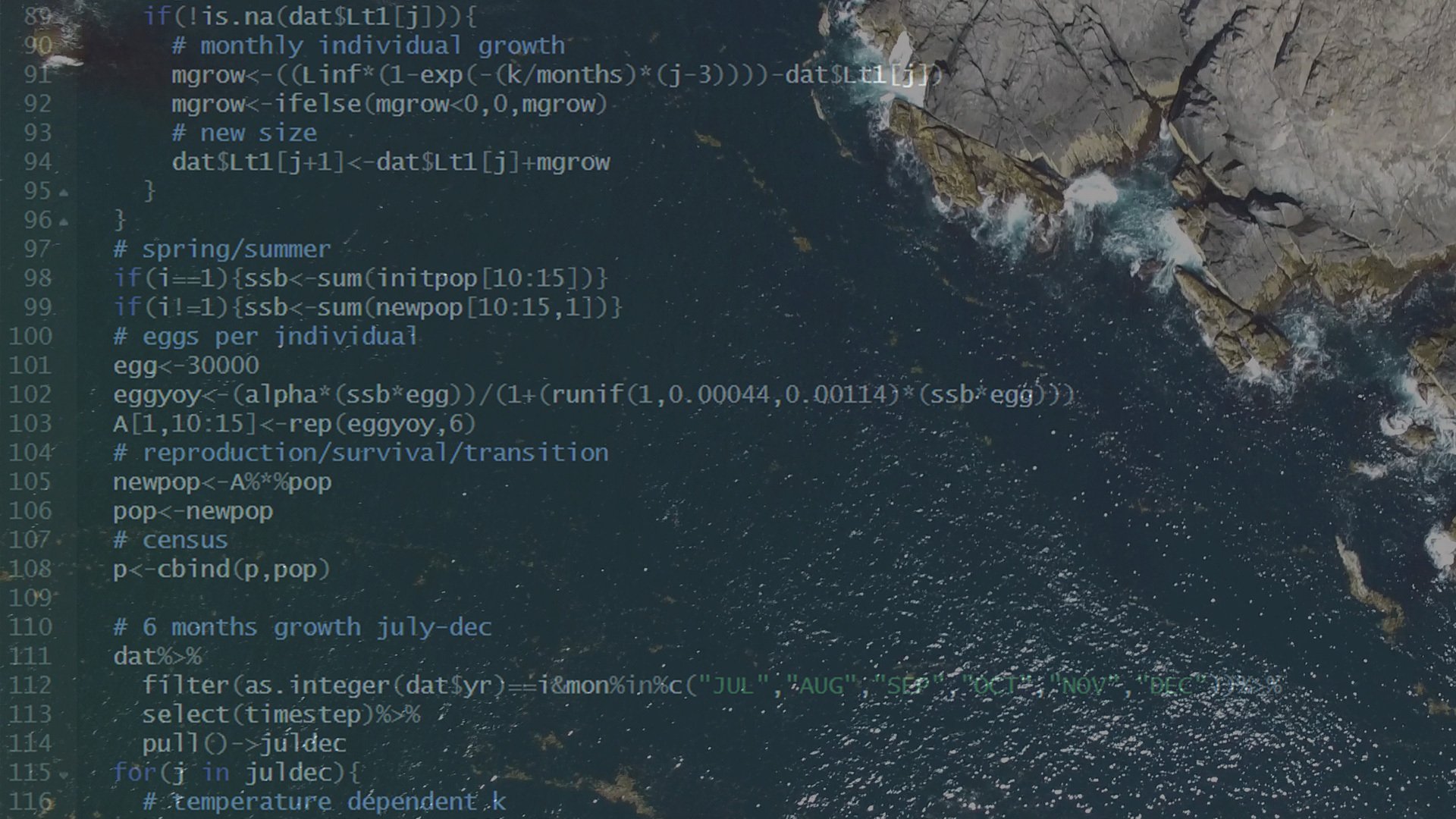
Our ever-changing world is complex, random, and chaotic — and the responsibility of making sense of it all often falls on the shoulders of scientists. Across many academic disciplines and economic sectors, computer modeling has emerged as one of the most valuable tools available to these scientists as they seek to create meaning out of messy datasets. For example, we know when and where hurricanes are coming and how strong they’ll be because meteorologists model storm paths and intensities. Models like those used during the COVID-19 pandemic project the spread of infectious diseases, which informs those making public health decisions. Financial models predict market trends, helping investors make informed decisions and manage risks.
Here at the Gulf of Maine Research Institute, one of the issues we are tackling is ensuring that the Gulf of Maine ecosystems and the communities that depend on them can continue to thrive in a warming world — and to help us do that, we also lean on the power of modeling.
One fish, two fish, where are you fish?
Dr. Andrew Allyn, a spatial modeler in Dr. Kathy Mills’ Integrated Systems Ecology Lab, has always been an avid fisherman. Even as a kid, he was in tune with where the fish were. But even in his favorite spots, he noticed that while sometimes he caught one after another, other times he couldn't get a single bite. This conundrum fascinated him. If he could only know with a little bit more certainty where the fish might be and when, he’d have a lot more successful fishing days. While the ocean is a bit bigger than his childhood fishing spots, Andrew points out that commercial fishermen in the Gulf of Maine are faced with the same basic challenge — especially as warming temperatures continue to put species on the move. So too are fisheries managers, who need to set responsible catch limits based on species abundance. When it comes to the ocean, neither fishermen nor fisheries managers can afford to guess. That's why fisheries scientists like Andrew use models to project how fish populations will shift as environmental conditions, predator and prey distributions, and biological life cycles change.
Today, Andrew is a Senior Research Manager trying to pin down where fish are in the Gulf of Maine to support both fishermen and fisheries managers. In his current work, Andrew relies on data collected by fisheries surveys — ranging from North Carolina to Nova Scotia, and covering inshore and offshore waters. These surveys provide location information for many marine species, spanning a large spatial area and long time period. Andrew and his team then relate this information to environmental conditions using computer models, allowing them to project where these species might move to in the future based on expected temperature changes. As a final step, Andrew works closely with our Integrated Systems Ecology Lab, Climate Center, and Community teams to make sure that this information is passed along to fishermen and resource managers to help them plan for and adapt to potential future conditions.
"I got into this work because I loved being outside, trying to make sense of the natural world. Although now I spend a lot of time inside behind a computer, I’ve learned to love the data analysis and modeling process, too. Models allow us to test our understanding about the patterns we see in the natural world, and to relay that insight to decision-makers.
Andrew Allyn, Ph.D. Senior Research Manager
The process of modeling where fish are and where they might be going is known as spatial modeling, and is critically important. However, it’s also important to understand which ecosystem conditions are most likely to affect fish populations, and the potential magnitude of those impacts. In the science world, this work is known as population dynamics modeling, and the information this method produces can help fishery managers understand threats and adjust management measures to try to sustain healthy populations and thriving fisheries.
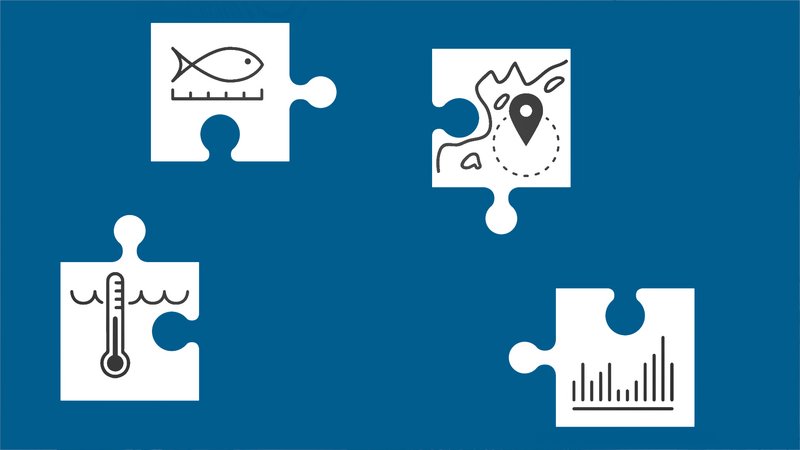
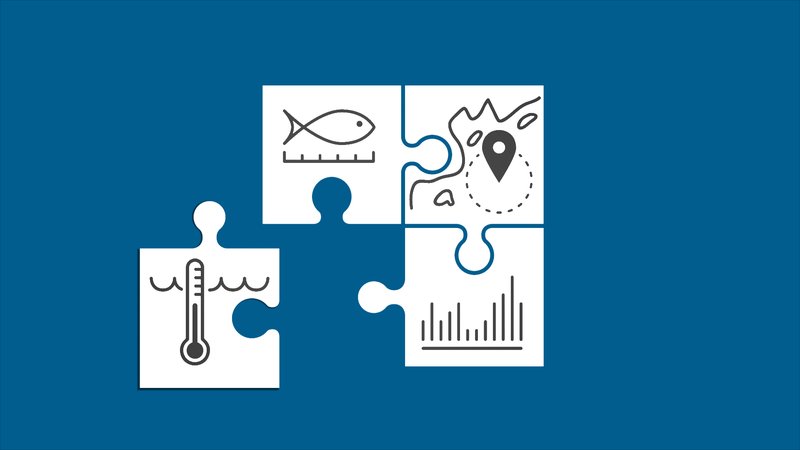
Miguel Barajas, a scientist at GMRI, uses computer models to study how environmental changes, such as ocean warming, affect fish populations. By experimenting with these models, Miguel can explore how different traits (e.g., the age at which fish mature, their growth rates, and their lifespan) influence their ability to survive in changing ecosystems. Some fish species may cope better with warmer oceans due to their life traits. For example, species that reproduce earlier and are more mobile might adapt by moving to cooler areas faster, while those that reproduce later may have more stable populations because their young have a higher survival rate despite temperature changes.
To break this down, think of smaller species like mice versus large animals like whales. Mice are small, and can have a high quantity of offspring early in their lifespans. They are also quick and mobile, and can move to different locations hastily if they need to find more suitable habitat. On the other hand, whales grow slowly, and become quite large before they reproduce. They focus more on the quality of their offspring instead of quantity, which tends to give their young a higher survival rate — even if conditions change.
Miguel's models show how these kinds of life history traits can help or hinder a population's ability to thrive in new or worsening conditions. These findings can help identify which species are most sensitive to environmental changes and how they might respond, aiding managers in adjusting conservation efforts accordingly. As the ocean warms, fish will either need to adapt to the heat or move to cooler waters. By simulating population changes under different warming scenarios, we can understand these interactions better, leading to smarter decisions in fisheries management. This approach helps evaluate assumptions, refine questions, and provide deeper insights. Ultimately, by improving our understanding of fish population dynamics, Miguel’s research helps us anticipate and adapt to changes in fish populations and ecosystems.
"The vastness of the ocean makes it both fascinating and challenging to examine. Developing simulation models based on the current understanding of ecosystem dynamics and life history theory allows me to test hypotheses for processes that are difficult to observe directly.
Miguel Barajas Quantitative Research Associate
Projections versus Predictions
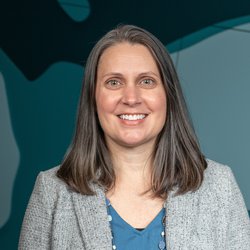
In the news and other popular media, the words "projection" and "prediction" are often used interchangeably. In a scientific context, the differences matter.
Projections
When scientists make projections, they are exploring what might happen based on a range of conditions that might occur in the future. Those future conditions are not known with certainty but can be characterized using ‘what if’ scenarios. What if we cut down our greenhouse gas emissions from the current level? Then we can project that temperatures will only rise X amount.
Predictions
When scientists make predictions, they are using information about current conditions to say what will likely happen in the future. For example, scientists can predict tomorrow’s weather quite reliably based on atmospheric conditions today.
Bringing Modeling to Classrooms
Another important piece of the work we do here at GMRI is teaching future generations how to think critically and solve complex problems using models, by working with teachers to bring modeling into classrooms.
Dr. Amanda Dickes, who leads our Learning Sciences Lab, recently collaborated with nine middle school teachers in Maine to explore classroom practices that expand students' understanding of modeling in science. Their goal was to introduce middle-school students to critical knowledge building practices through the construction and modification of scientific models that show changes in relevant local ecosystems and species — such as green crabs in the intertidal zone, or the spread of Japanese knotweed in local forests. The students ventured out in the field to collect data from these local ecosystems. Back in the classroom, the students used their observations from the natural world to construct their own models, which they then iteratively revised to test complex ecosystem questions of their own choosing.
In addition to creating first-hand ecosystem modeling experiences for students, Amanda and her team also study how teachers and students develop these concepts and practices in a classroom setting. The results of this research inform the design of more effective curriculum resources.
All models are wrong, but some models are useful
Think of a model like a map. A map isn't a perfect copy of the real world — it's a simplified version that helps us understand where things are. If a mapmaker tried to include every detail, the map would be impossible to use. Similarly, data models are simplified versions of reality that help us make sense of complex things. While no model can capture every detail perfectly, a good model gives us just enough information to make smart decisions. So, even though all models are 'wrong' in the sense that they aren't exact, they can still be incredibly useful tools for understanding and solving problems. Without models, we would not be able to ensure that the Gulf of Maine ecosystem and the communities that depend on it continue to thrive in a warming world.